Numbers are the highest degree of Knowledge. It is the knowledge itself. — Plato Poster.
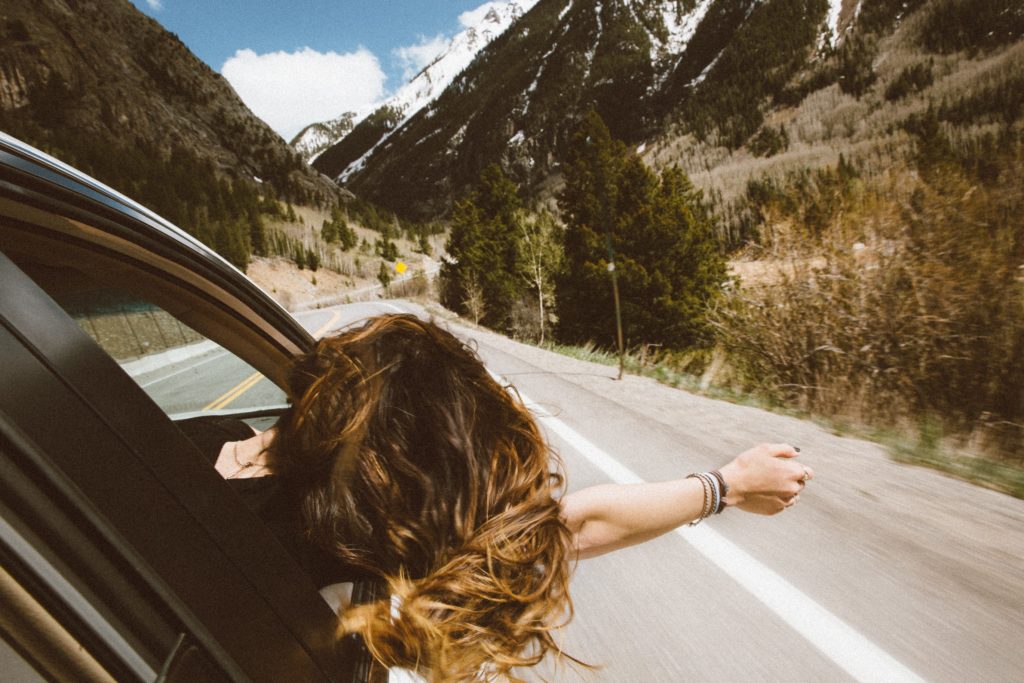
Here is my understanding of Degrees Of Freedom.
Degrees of freedom refers to the maximum number of logically independent values in a data sample which have the freedom to vary within.
Example:
If there is a sample of 3 values {5, x, 15} and the mean of all the values is 10.
Now it is easy to say that the value of x would be 10 as the mean of these 3 values is 10.
But if 2 values from this sample are not known, say {5, x, y} with the same mean 10, then we cannot be sure about the exact values of x & y.
It could be any values from (10, 15), (15, 10), (5, 20), (20, 5) or even (1, 24).
So we cannot find the exact value of these data x & y.
These 2 values have the freedom to vary.
But the third value does not have the freedom to change as it should be some value so that the mean will not change. So this value depends upon all the other values.
So the degrees of freedom of this sample data of size 3 is 2.
Not only with size 3 sample, a sample with any size we can find only one value if it is unknown as it depends on all the other values in the sample.
So the degrees of freedom are always the sample size minus 1.
Formula:
V = n — 1
V = Degrees of freedom
n = Sample size
In the above example, there is only one constraint placed in the set that the “mean is 10”.
Therefore the constraint placed on freedom is one and degrees of freedom is two.
If we mention the number of constraints as k, then
V = n — k
As the restrictions increase, freedom is reduced.
In the above matrix of 2 X 2, the degrees of freedom of Gender and Result, each having 1 constraint in it (Total) and size is 2, then the degrees of freedom is as given below:
V (nu) = (c — 1) (r — 1)
= (2–1) (2–1)
= 1
P.S. Contingency Table: In statistics, a contingency table (also known as a cross tabulation or cross-tab, Pivot table that we use in Excel) is a type of table in a matrix format that displays the (multivariate) frequency distribution of the variables.
Conclusion:
Degrees of freedom of a data set is n — k, whereas n — Size of data set & k — Number of constraints placed.
As the constraints increase, freedom is reduced.
If there is more than one variable are combined into a matrix, then the entire degrees of freedom is the product of degrees of freedom of each variable.